Artificial Intelligence (AI) in healthcare makes patient care more personalized, efficient, and proactive. AI trends in healthcare include early diagnosis through AI-enabled systems, automation of administrative tasks with AI-powered tools, and better treatment planning using predictive analytics. As healthcare systems face rising costs and staff shortages, AI plays a growing role in improving outcomes.
The global AI in healthcare market is set to grow from USD 36.96 billion in 2025 to USD 613.81 billion by 2034, with a compound annual growth rate (CAGR) of 36.83%. This article explores 10 key AI trends reshaping healthcare and what they mean for healthcare businesses and individuals.
What are the Top 10 AI Trends in Healthcare?
- Conversational AI and Agentic AI
- Personalized Healthcare
- AI-powered Imaging and Diagnostics
- AI Governance and Responsible AI
- Patient Management
- Predictive Analytics
- AI for Mental Health
- AI-enabled Robotics
- Public Health Management
- Administrative Automation
Methodology: How We Created the Healthcare AI Trend Report
For our trend reports, we leverage our proprietary StartUs Insights Discovery Platform, covering 7M+ global startups, 20K technologies & trends, plus 150M+ patents, news articles, and market reports.
Creating a report involves approximately 40 hours of analysis. We evaluate our own startup data and complement these insights with external research, including industry reports, news articles, and market analyses. This process enables us to identify the most impactful and innovative trends in the healthcare industry.
For each trend, we select two exemplary startups that meet the following criteria:
- Relevance: Their product, technology, or solution aligns with the trend.
- Founding Year: Established between 2020 and 2025.
- Company Size: A maximum of 200 employees.
- Location: Specific geographic considerations.
This approach ensures our reports provide reliable, actionable insights into the healthcare AI innovation ecosystem while highlighting startups driving technological advancements in the industry.
Innovation Map outlines the Top 10 AI Trends in Healthcare & 20 Promising Startups
For this in-depth research on the Top AI Trends & Startups in Healthcare, we analyzed a sample of 3090 global startups & scaleups. The Healthcare AI Innovation Map, created from this data-driven research, helps you improve strategic decision-making by giving you a comprehensive overview of the AI trends & startups in healthcare that impact your company.
Tree Map reveals the Impact of the Top 10 AI Trends in Healthcare
The AI in Healthcare Tree Map highlights the Top 10 Healthcare AI Trends in 2025. These include clinical AI agents, call assistants, precision medicine, data integration, and harmonization AI platforms.
The market also adopts deep neural networks, AI-powered public health platforms, humanoid robots, digital therapists, and more. These trends reflect how the market leverages AI technologies to improve care delivery and population health outcomes across healthcare systems.
Global Startup Heat Map covers 3090 AI Startups & Scaleups in Healthcare
The Global Startup Heat Map showcases the distribution of 3090 exemplary startups and scaleups analyzed using the StartUs Insights Discovery Platform. It highlights high startup activity in the US and India, followed by the UK and Canada. From these, 20 promising startups are featured below, selected based on factors like founding year, location, and funding.
Want to Explore Healthcare AI Innovations & Trends?
Top 10 Emerging AI Trends in Healthcare [2025 and Beyond]
1. Conversational AI & Agentic AI
Conversational AI tools, such as chatbots and virtual assistants, deliver continuous access to health information, schedule appointments, and check symptoms. They increase patient engagement and reduce the workload on healthcare staff. Pfizer‘s Medibot, for instance, answers medication and treatment queries while streamlining communication and raising patient satisfaction.
Moreover, these conversational AI systems automate routine tasks such as appointment scheduling, billing inquiries, and patient data collection. This frees up healthcare professionals to focus on patient care.
During the COVID-19 pandemic, Translators Without Borders (TWD) deployed a multilingual chatbot to deliver accurate health information in Congo and Nigeria. This directly counters misinformation.
The conversational AI in the healthcare market was valued at USD 13.53 billion in 2024 and is expected to reach USD 48.87 billion by 2030, rising at a CAGR of 23.84%.
Further, agentic AI systems autonomously manage intricate tasks like medical coding, diagnostics, and treatment planning. NYUTron analyzes patient data and suggests treatment options to support real-time clinical decisions. It also combats misinformation directly through evidence-based recommendations.
Driven by these innovations, the global agentic AI market in healthcare is set to witness a growth rate of 35-40% in the next five years (2025-2030).
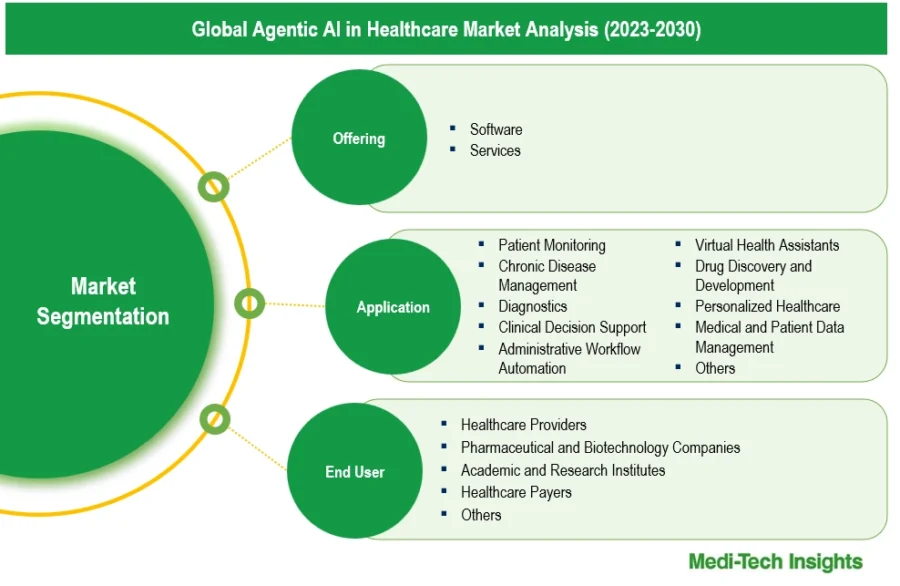
Source: Medi-Tech Insights
Natural language processing (NLP) and understanding (NLU) technologies enable AI systems to comprehend and process human language. This facilitates effective communication between patients and healthcare providers.
Additionally, generative AI creates human-like responses tailored to individual patient needs to improve the quality of virtual consultations.
Voice-enabled virtual assistants also allow hands-free interaction. This aids in appointment scheduling and symptom checking. For example, Microsoft‘s Dragon Copilot assists healthcare professionals by automating clinical documentation through voice dictation.
Further, automated medical coding and billing systems streamline the coding process and reduce errors and administrative workload.
Jivi Health builds Clinical AI Agents
Jivi Health is an Indian startup that develops AI-powered medical agents that autonomously support clinical diagnosis, treatment planning, and health management.
Built on an agentic AI architecture, its conversational platform integrates voice, imaging, and patient history to generate real-time, personalized medical insights. Moreover, the platform includes specialized agents like the symptom agent for triage, a risk agent for disease prediction, and an auto-scribe agent for clinical documentation.
Additionally, these agents operate from emergency response to preventive care to improve decision-making and reduce administrative workload. This integration improves healthcare delivery by providing consistent, efficient, and accessible services.
Inquira Health deploys AI-powered Call Assistants
Dutch startup Inquira Health offers AI-powered voice assistants that automate administrative tasks in healthcare operations. It also has a conversational AI platform that improves call scripts into interactive workflows. This enables automated appointment scheduling, patient intake, follow-up calls, and medication reminders through voice interactions.
Moreover, the platform supports multiple languages, offers customizable voice tones, and integrates with healthcare standards via REST and FHIR application program interfaces (APIs). It also provides real-time transcripts, performance analytics, and compliance monitoring.
2. Personalized Healthcare
Personalized medicine considers individual variability to deliver more accurate diagnoses and effective treatments. For instance, pharmacogenomics enables clinicians to predict how patients respond to specific medications to reduce adverse drug reactions and increase treatment efficacy.
Genomics underpins personalized healthcare by allowing healthcare providers to identify genetic mutations that affect disease and treatment response. Advances in sequencing technologies and efficiency are dropping the cost of whole-genome sequencing (WGS) to as low as USD 600 in some cases. This cost reduction drives the broader adoption of personalized healthcare in clinical settings and makes it more accessible to a wider population.
Healthcare providers are integrating personalized medicine into routine clinical practice by leveraging genomic insights to tailor prevention, diagnosis, and treatment strategies. This shift contributes to the rapid growth of the global personalized medicine market, which is projected to expand from USD 654.46 billion in 2025 to approximately USD 1.31 trillion by 2034. The market size is expanding at a CAGR of 8.10% from 2025 to 2034.
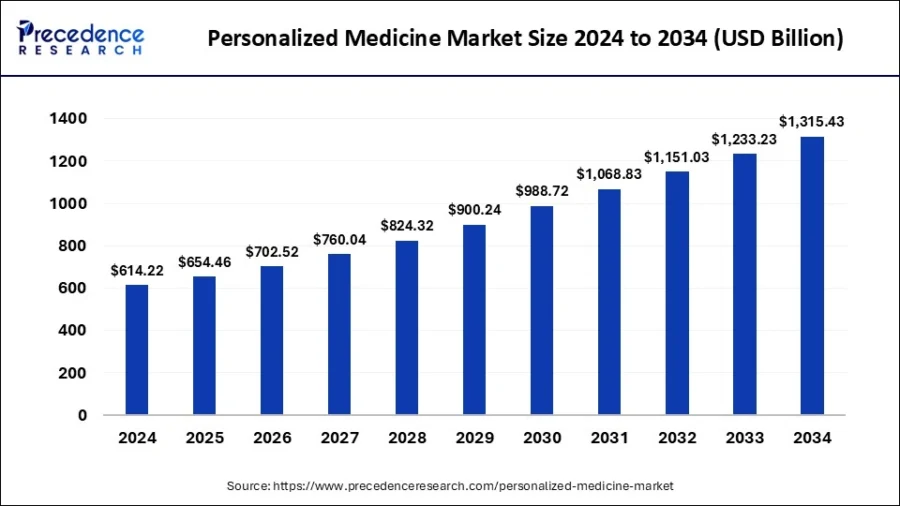
Source: Precedence Research
AI-powered clinical decision support systems (CDSS) analyze vast datasets, including patient records and medical literature. These systems assist clinicians in making informed decisions. For instance, Tempus AI utilizes AI to provide precision diagnostics and treatment recommendations in oncology, cardiology, and depression care.
Further, AI is improving personalized healthcare by analyzing vast datasets to tailor treatments. In India, IIT Madras developed Garbhini-GA2, an AI model that provides accurate fetal age estimations for Indian women.
Additionally, HERMES kiosks utilize AI to deliver personalized over-the-counter medication recommendations in public spaces. These kiosks analyze patients’ symptoms and medical histories to offer personalized over-the-counter medication recommendations while preserving privacy through federated learning.
Telemedicine platforms are also incorporating AI to predict health deterioration and personalize care. The PrediHealth project combines telemonitoring with AI to manage chronic heart failures, which reduce hospitalizations and improve patient engagement.
Companies like Nourish leverage AI to provide personalized nutritional guidance. Such tools connect patients with dietitians for virtual consultations and support patients on weight-loss medications by offering tailored nutrition plans.
Horus ML specializes in Precision Medicine
Spanish startup Horus ML develops AI-powered solutions that enable personalized healthcare across diagnostic imaging, medicine, remote monitoring, and mental health management.
The precision healthcare tools encompass early detection, clinical decision support, and individualized care planning through predictive analytics and patient-specific insights. Moreover, the machine learning-based tool AI4TeenDep identifies depressive patterns in adolescents using executive function scales and speech analysis.
Mental Health Triage is an AI-powered tool that stratifies patients based on severity and clinical needs to prioritize care delivery in mental health services. Further, Horus Predictor is a solution based on machine learning models that uses admission data to estimate hospital stay duration and readmission risk.
Open Health offers a Data Integration and Harmonization AI Platform
Dutch startup Open Health delivers a unified digital platform that integrates and interprets laboratory test data using AI. The platform ingests lab results from API or PDF, harmonizes them into a standardized structure, and enriches the data using proprietary medical intelligence.
Moreover, this process enables clear, user-friendly visualizations and personalized digital reports that reflect the branding of partner laboratories. The platform also provides a secure portal for patients to access their complete test history.
3. AI-powered Imaging & Diagnostics
AI-powered diagnostics bridge healthcare gaps in areas with limited medical resources. An AI-enabled chest X-ray system deployed across 17 Indian healthcare facilities processed over 150K scans, averaging 2000 daily. This deployment reduces reporting times and improves diagnostic accuracy, particularly in underserved regions.
AI algorithms also detect subtle patterns in medical images to improve diagnostic precision. A collaboration between Massachusetts General Hospital and MIT developed an AI system that detects lung nodules with 94% accuracy, surpassing the 65% accuracy rate of human radiologists. This advancement improves early lung cancer detection and patient outcomes.
Moreover, AI streamlines diagnostic processes by reducing time and workload for healthcare professionals. The UK’s National Health Service (NHS) implements an AI tool, DERM, to speed up skin cancer diagnostics. This tool utilizes smartphone images to assess skin lesions and delivers results in approximately five minutes. Clinical evaluations demonstrate that DERM achieves a 99.9% accuracy rate in ruling out melanoma – the most severe form of skin cancer.
The global AI in diagnostics market is projected to grow to USD 12.65 billion by 2034, rising at a CAGR of 24.64% from 2024 to 2034.
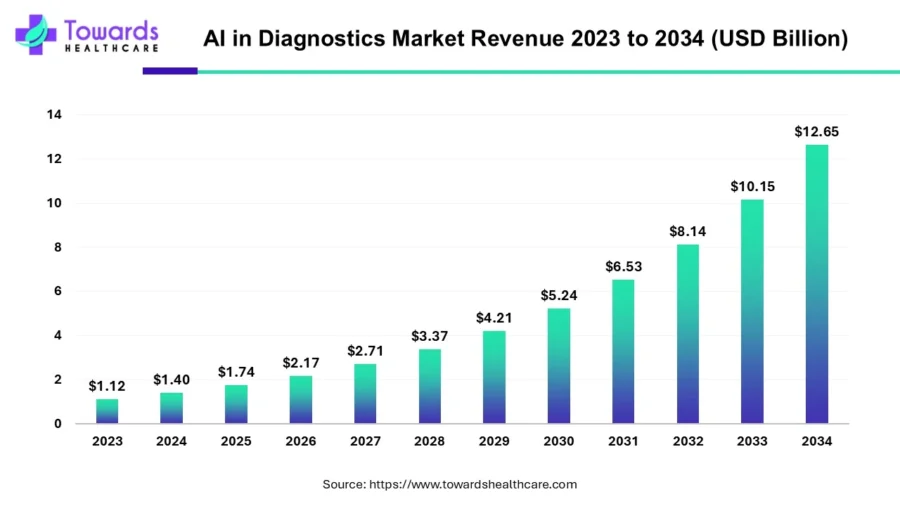
Source: Towards Healthcare
Additionally, researchers developed a deep learning model trained on 2 million MRI spine scans that detects 43 spinal pathologies with 97.9% accuracy. This improves diagnostic efficiency and reduces reporting times.
AI-enhanced ultrasound imaging system incorporates real-time image processing, organ tracking, and voice commands to increase diagnostic efficiency. The system achieves 98.6% accuracy in detecting liver fibrosis. Further, Google‘s collaboration with Apollo Radiology International leverages AI to detect diseases like tuberculosis, lung, and breast cancer early.
Ainovis offers Medical Images as a Service
Spanish startup Ainovis develops generative AI technology that produces synthetic medical images to support diagnostic model training. Its ANV-GEN platform generates realistic MRI and CT scans across anatomical regions by extrapolating from minimal real-world data. This enables the creation of datasets without compromising patient privacy.
Moreover, the platform simulates a wide range of pathologies and imaging modalities to improve the generalizability of AI models. Additionally, the ANV-SR tool refines image quality by emphasizing subtle details to improve the clarity of underperforming scans.
AIGEA Medical develops a Deep Neural Network
AIGEA Medical is an Italian startup that offers DeepMammo, an AI-driven cloud platform assisting radiologists in early breast cancer detection. It employs proprietary generative AI algorithms and integrates multimodal neural networks with explainable AI techniques to analyze digital mammography images.
By automating repetitive tasks and highlighting areas of concern, DeepMammo reduces screening time and improves diagnostic accuracy. Its explainable AI components further provide transparency in decision-making and increase trust among clinicians.
Additionally, DeepMammo supports digital imaging workflows to increase radiologists’ performance, improve diagnosis accuracy, decrease daily workloads, and reduce costs.
4. AI Governance & Responsible AI
AI models trained mostly on men of European descent may misdiagnose or provide less effective treatments for women and other ethnic groups. To address algorithmic bias, developers are building responsible AI frameworks to detect and correct these biases. This ensures fair and accurate healthcare recommendations for all.
Moreover, transparency in AI decision-making processes is vital for building trust among healthcare professionals and patients. By implementing explainable AI models, healthcare professionals and patients identify and validate AI-driven recommendations.
Healthcare AI systems handle sensitive patient data, which makes data governance essential. Therefore, responsible AI practices enforce strict data privacy measures, ensure compliance with regulations, and protect patient confidentiality. Solutions like explainable AI (XAI) frameworks improve transparency by making AI decision-making processes interpretable for clinicians and patients.
AI governance and responsible AI thus increase trust and facilitate informed decision-making in clinical settings. Google‘s Responsible AI Progress Report outlines efforts in implementing governance structures throughout the AI development lifecycle. This report focuses on risk management and ethical considerations in healthcare applications.
For instance, Mayo Clinic integrates explainable AI models into its radiology department. These models provide transparent insights into AI-driven analyses of medical images and allow radiologists to better understand and validate the AI’s conclusions.
Additionally, healthcare organizations are implementing tools that audit AI models for fairness to mitigate algorithmic bias, especially against underrepresented groups. Such tools assess training data and model outputs to identify and correct disparities. The Brookings Institution‘s AI Equity Lab develops interdisciplinary approaches to designing inclusive AI technologies in healthcare to address disparities and promote equitable access.
Further, patient consent management systems allow patients to control their data usage in AI systems. This further increases transparency and trust. Federated learning models also enable collaborative model training across multiple institutions without sharing sensitive patient data to preserve privacy while increasing model resilience.
Given these advances and the growing emphasis on ethical and transparent AI practices, the demand for AI governance solutions is improving rapidly. The global AI governance market size is projected to grow from USD 0.84 billion in 2025 to USD 26.91 billion by 2035, representing a CAGR of 41.36%, during the forecast period 2025-2035.
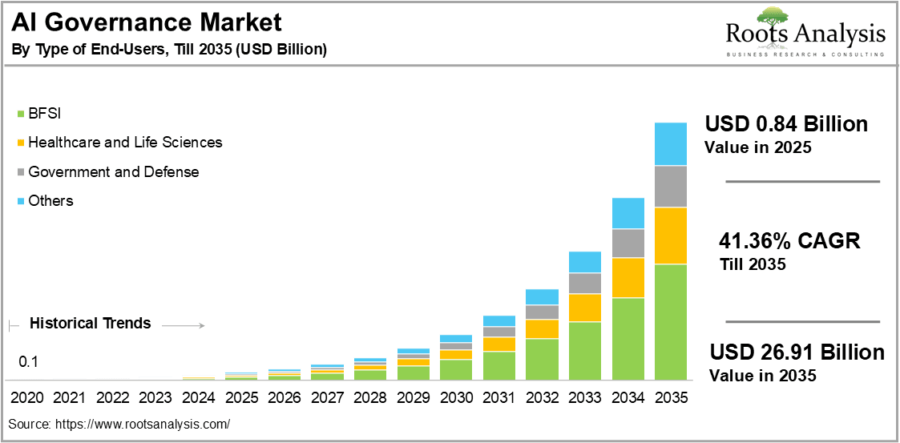
Source: Roots Analysis
VerioHealth.AI specializes in Responsible AI
VerioHealth.AI is a US-based startup that develops an AI governance platform that facilitates the responsible and ethical deployment of AI in healthcare. It follows a structured lifecycle approach and conducts pre-deployment evaluations to ensure safety, reliability, and performance benchmarking.
Moreover, the platform adapts deployments to align with local workflows and post-deployment to detect model drifts and recalibrate systems. It also enforces continuous governance to maintain compliance, equity, and transparency. Additionally, the platform incorporates tailored risk management processes to detect and mitigate biases, security flaws, and inaccuracies early.
Its performance evaluation framework guarantees that AI models meet strict reliability, fairness, and accuracy standards. Further, the platform delivers ongoing oversight to maintain ethical AI operations and proactively addresses patient safety and equity issues across diverse populations.
Hippocraticum AI provides a Clinical Documentation Platform
Hippocraticum AI is an Italian startup that provides a locally deployable AI assistant that streamlines clinical documentation and delivers actionable patient insights. Its proprietary models operate within the healthcare provider’s infrastructure and mitigate the transmission of patient data to external servers or third parties.
By analyzing available patient information, the AI assistant offers clinicians decision-support tools that improve diagnostic accuracy and save time. It also aligns with emerging global standards for responsible AI in healthcare while emphasizing transparency, accountability, and data sovereignty.
5. Patient Management
Patient management systems (PMS) streamline administrative tasks, like appointment scheduling, billing, and patient tracking, to save time and costs. The implementation of electronic medical records (EMR) in Indonesian health facilities reduced patient waiting times by 15-20 minutes and service duration by 20-25 minutes.
Modern PMS integrated with customer relationship management (CRM) tools actively increases patient engagement and satisfaction. These tools automate appointment reminders, streamline communication, and provide patients with easy access to their medical records. Personalized interactions and efficient information management also allow healthcare providers to deliver more responsive and patient-centered care.
AI-powered appointment scheduling systems automate appointment booking based on doctor availability, urgency, and patient preferences, while patient triage chatbots conduct pre-visit assessments, triage patients to appropriate care pathways, and gather clinical history.
Further, AI integration in PMS enables predictive analytics for patient care and optimizes workflows. PMS also supports remote patient monitoring (RPM) by enabling continuous monitoring of patients outside clinical settings. This is crucial for chronic disease management and post-hospitalization care.
This growing adoption of intelligent, integrated systems signals a broader industry shift toward proactive, data-driven patient care. As per InsightAce Analytic, the AI market in patient management is predicted to witness a 24.51% CAGR during the forecast period from 2023 to 2031.
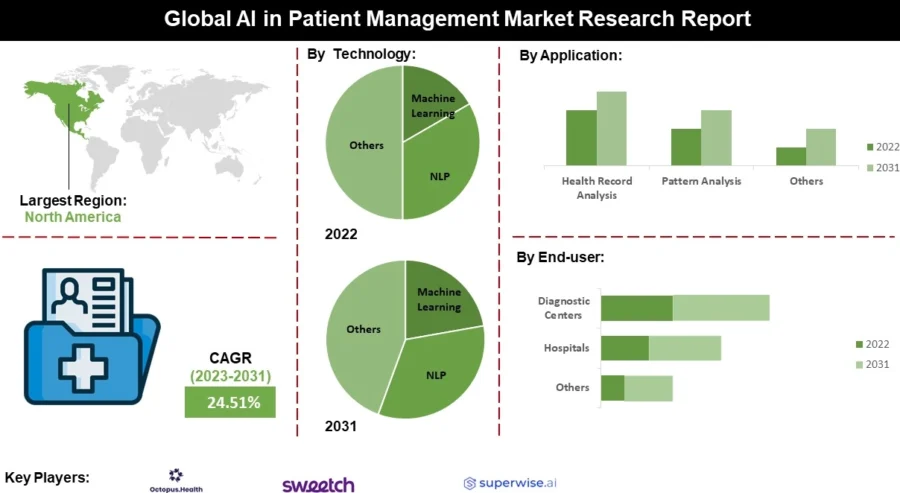
Source: InsightAce Analytic
Sumo Analytics builds a Patient Flow Optimization System
Spanish startup Sumo Analytics develops ALZA CARE, an AI-driven platform that improves hospital operations by forecasting patient flow and optimizing resource allocation. The platform integrates with existing hospital infrastructure to analyze real-time and historical data. This enables precise predictions of patient admissions, discharges, and readmission risks.
Moreover, ALZA CARE streamlines bed assignments, staff scheduling, and equipment utilization. This reduces emergency department overcrowding and minimizes elective surgery cancellations.
TouchPoints.health develops a Patient Management SaaS Platform
TouchPoints.health is a UK-based startup that offers a secure, cloud-based medical practice management platform. It integrates real-time outcome reporting with personalized, time-relevant patient communications.
The platform enables clinicians to track patient progress and detect deviations from expected recovery milestones. It captures and analyzes patient interactions across channels, including SMS, email, and app-based questionnaires. Clinicians curate touchpoints tailored to each patient’s treatment plan and offer timely engagement and adherence to care protocols.
The platform’s customizable architecture allows healthcare providers to adapt its features to their specific operational needs. Further, TouchPoints.health reduces administrative burdens, improves patient engagement, and supports proactive clinical decision-making.
6. Predictive Analytics
Predictive models analyze patient data to identify the risk of developing conditions, like diabetes, heart disease, and cancer, for timely interventions. Healthcare providers analyze patient data to tailor treatments that increase effectiveness, minimize side effects, and improve patient outcomes. Moreover, predictive analytics forecast patient admissions, optimize staffing, and manage resources, leading to major cost savings.
This growing reliance on data-driven strategies is improving the healthcare predictive analytics market. The global healthcare predictive analytics market size is projected to grow from USD 16.75 billion in 2024 to USD 184.58 billion by 2032, exhibiting a CAGR of 35.0%.
Machine learning algorithms analyze extensive patient data to identify individuals at risk of developing conditions such as diabetes, heart disease, and cancer. The UK’s National Health Service (NHS) initiated a trial of the Aire-DM tool, which uses AI to predict type 2 diabetes risk up to 13 years in advance by analyzing ECG readings.
AI models also tailor treatments based on individual patient data to improve efficacy and reduce side effects. A noteworthy example is Quibim‘s QP-Prostate software. It is developed in collaboration with Philips, which uses AI to improve prostate cancer diagnosis through MRI analysis.
Additionally, AI-powered devices monitor patients’ vital signs remotely and enable early intervention. Philips‘ 2024 future health index report indicates that 41% of healthcare leaders plan to invest in AI for remote patient monitoring over the next three years. AI also improves drug discovery and optimizes clinical trials by predicting outcomes and identifying suitable participants.
Further, AI analyzes health data across populations to identify trends and inform public health strategies. The CDC‘s updated public health data strategy for 2024-2025 provides timely and actionable data to improve health outcomes.
Afya Intelligence creates AI-powered Public Health Platforms
Afya Intelligence is a Tanzanian startup that develops AI-driven predictive analytics platforms to improve healthcare decision-making. Its product, Afyalytics, integrates data from systems like DHIS2 and eLMIS to automate data cleaning, visualization, and forecasting. This enables healthcare providers to anticipate supply chain needs and patient demand.
Moreover, the platform offers customizable dashboards and quick analysis tools. It also simplifies complex datasets for users with varying data proficiency levels. This way, Afya Intelligence delivers actionable insights that improve accessibility and enable healthcare providers to make timely, evidence-based decisions.
Percipio Health provides a Daily Health Monitoring Platform
Percipio Health is a US-based startup that offers an AI-powered population health monitoring platform. It leverages smartphone-based data collection to assess and manage health risks for diverse patient populations.
The platform also operates through a mobile application, which gathers multiple health signals daily. This includes vital signs via facial scans, cognitive assessments through voice recordings, and medication adherence through photo journaling.
Additionally, the platform analyzes these inputs through multi-signal analysis. This enables clinicians to receive predictive insights and recommended clinical actions tailored to individual patient profiles. Its device-free method allows healthcare providers and payers to extend care to rising and high-risk populations efficiently.
7. AI for Mental Health
AI-powered chatbots and virtual assistants provide immediate and cost-effective mental health support, especially in regions with limited access to professionals. Platforms, like Wysa and Woebot, offer continuous conversational support. These platforms bridge the treatment gap in underserved areas.
AI systems effectively analyze patient data and tailor interventions based on individual needs. For instance, Spring Health employs machine learning algorithms to match patients with appropriate therapy modalities. These systems improve treatment efficacy and reduce trial-and-error in healthcare.
Moreover, AI identifies early signs of mental health issues by analyzing speech patterns, facial expressions, and online behavior. Tools like Cogito utilize voice analysis to assess emotional states to enable timely interventions before conditions escalate. AI also assists in administrative tasks and preliminary assessments, allowing mental health professionals to focus on complex cases.
AI companions also provide emotional support, particularly for the elderly experiencing loneliness. For instance, InTouch AI Companion offers daily calls to engage in meaningful conversations and monitor emotional well-being. Further, AI-assisted crisis support tools offer immediate assistance during mental health crises.
Further, conversational AI chatbots offer continuous mental health support. These chatbots make them especially valuable in areas with limited access to mental health professionals. The EmoBay platform provides 24/7 conversational support, mood tracking, and crisis-response guidance.
According to Towards Healthcare, the global AI in mental health market size is calculated at USD 1.80 billion in 2025 and is expected to reach around USD 11.84 billion by 2034, growing at a CAGR of 24.15% for the forecast period.
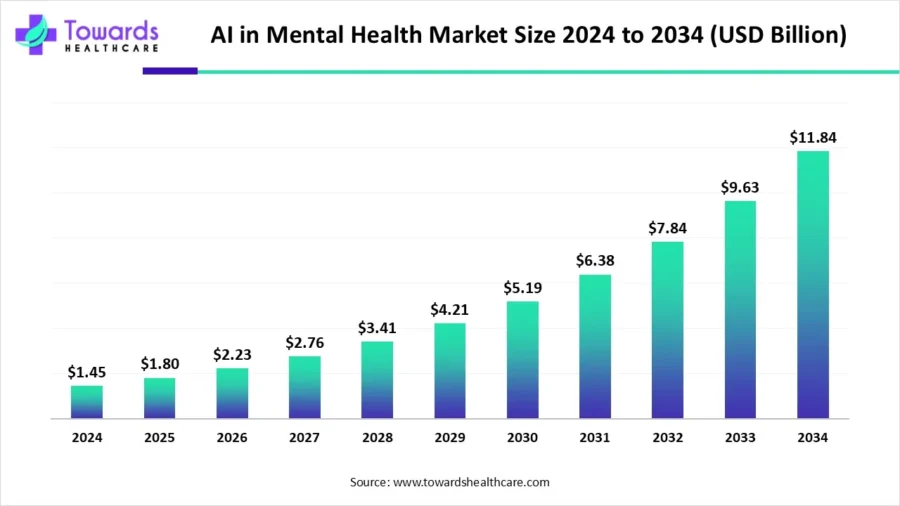
Source: Towards Healthcare
UpLift Ai builds an AI Co-Therapist
UpLift Ai is an Australian startup that develops an AI-powered platform for mental health clinics. It automates clinical workflows, improves patient engagement, and supports therapists through continuous, data-informed care. The platform integrates AI-driven tools for scheduling, clinical documentation, treatment planning, and reporting.
It also provides real-time patient risk alerts and behavioral insights to support proactive decision-making. Further, the platform features an AI co-therapist, Astra, that offers continuous therapeutic interactions between sessions to maintain momentum and reduce dropout rates.
NoSuffering offers a Digital Therapist Application
UK-based startup NoSuffering provides Cerina, a digital mental health application that delivers AI-powered, cognitive-behavioral therapy (CBT) through a mobile application. The app guides through structured CBT modules, including tools like a worry diary, symptom tracker, and therapy logs.
Cerina integrates features like an AI chatbot for real-time emotional support, multilingual content, and psychoeducational resources. Moreover, it employs adaptive algorithms to personalize therapy based on inputs and preferences.
8. AI-enabled Robotics
With global healthcare staffing challenges, AI-enabled robots handle routine tasks and free medical staff to focus on complex care. AI-powered robotic systems assist surgeons in performing complex procedures with greater accuracy. These robotic systems also reduce complications and offer faster patient recovery.
Further, AI-powered robots provide emotional support and assist with daily activities for the elderly. Cera Care deploys robots in the UK to assist in-home elderly care by reminding patients to take medications and connecting them with caregivers.
Similarly, in South Korea, approximately 10K Hyodol robots monitor medication adherence and detect emotional states. They improve the quality of care for seniors living alone. Robots also automate routine tasks like medication delivery and appointment scheduling. They provide more room for healthcare professionals to focus on patient care.
Currently, the global medical robots market size was valued at USD 12.8 billion in 2024 and is estimated to grow at 16.6% CAGR from 2025 to 2034.
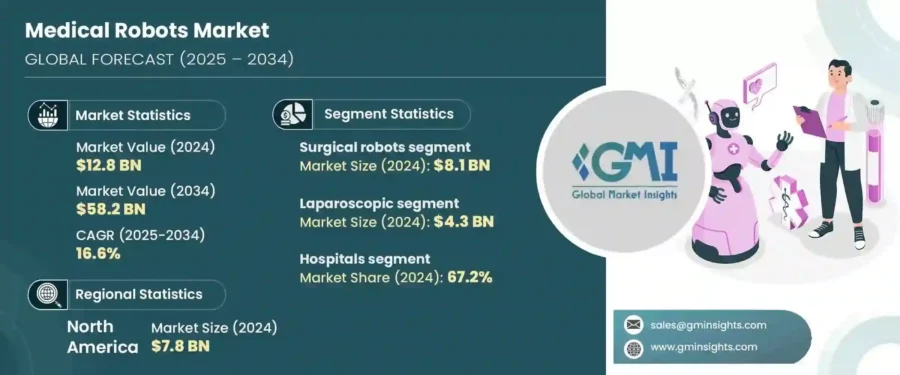
Source: GMI Insights
Robotic systems, like the da Vinci surgical system and CMR Surgical’s Versius, enable minimally invasive procedures with enhanced precision. They reduce complications and speed up patient recovery. Moreover, robots, like ABB’s mobile laboratory robot, automate routine tasks like medication delivery and appointment scheduling.
AI-powered virtual nursing assistants provide 24/7 support by answering patient queries, monitoring health status, and offering medication reminders. They also reduce hospital readmissions and alleviate the burden on healthcare staff. Additionally, AI integration in electronic health records (EHRs) facilitates better data management. This enables personalized treatment plans and improves patient outcomes.
AI-powered robotic exoskeletons further assist patients in rehabilitation by providing physical support and real-time feedback. These devices allow patients to regain mobility and independence after injuries or surgeries.
CAYTU Robotics provides Robotics-as-a-Service (RaaS)
Senegal-based startup CAYTU Robotics develops AI-enabled service robots and intelligent systems for healthcare applications. The company’s robot, Sito-E, navigates autonomously or through remote control within clinical environments. It also integrates real-time sensor data through Internet of Things (IoT) connectivity to interact with patients and staff.
Moreover, the startup’s robotics-as-a-service model allows hospitals to deploy scalable fleets without upfront capital investment. Its AI agents further automate routine workflows and support decision-making.
Enchanted Tools designs People-Facing Humanoid Robots
French startup Enchanted Tools offers Mirokai Explorer Suit, an AI-enabled humanoid robot to assist in healthcare environments. The robot integrates multiple large language models (LLMs) for natural language processing and vision-language models for contextual understanding.
Mirokai Explorer Suit also integrates LLMs for real-time speech recognition with emotional prosody to facilitate smooth human interaction. Moreover, the robot employs autonomous navigation using visual simultaneous localization and mapping (VSLAM) technology. This enables it to build a map of its environment while tracking its position using visual data.
It also utilizes AI-driven object manipulation for precise handling and incorporates GDPR-compliant face tracking to ensure safe and efficient operation in dynamic settings. Further, the humanoid robot features high-speed, torque-controlled actuators for precise and responsive movements. It also incorporates upgraded sensors for 360-degree environmental perception and optimized omnidirectional mobility.
9. Public Health Management
AI facilitates real-time monitoring of health data to enable swift responses to emerging public health threats. For example, the Epidemic-Climate dataset (EpiClim) integrates climate and health data across India. It allows AI models to predict outbreaks of diseases like dengue and malaria. These predictions support timely public health interventions.
AI also improves the ability to detect diseases early and predict health trends, enabling proactive interventions. The University of Pittsburgh partners with Amazon Web Services to establish the Health Sciences and Sports Analytics Cloud Innovation Center. It utilizes AI to monitor athlete health and predict injuries, showcasing AI’s role in preventive healthcare.
Solutions like climate-health predictive AI models integrate climate and health data to forecast disease outbreaks. AI-powered surveillance systems continuously monitor health signals from hospitals, clinics, and public reports. The World Health Organization (WHO) upgraded the Global Health Observatory (GHO) with AI surveillance tools to track respiratory and infectious diseases globally.
Moreover, AI-enhanced health event detection systems identify anomalies like sudden spikes in emergency room visits, drug sales, or symptom reporting. For example, BioSense, managed by the CDC, integrates AI to detect early signs of bioterrorism and pandemics by monitoring emergency room data.
AI further monitors mental health and behavior trends during crises via digital platforms. Koa Health’s AI mental health trend tracker monitors the population’s mental health post-lockdown using smartphone interaction patterns.
Kai-Xen offers an AI-powered Public Health Strategy Platform
Singaporean startup Kai-Xen develops an AI-powered platform that converts real-time population health data into actionable public health strategies. It integrates genetic profiles, lifestyle patterns, and geospatial data into predictive models that support disease risk stratification and precision policymaking.
Moreover, the startup’s public health decision support system enables policymakers to check and implement data-driven interventions for specific population needs. This ensures strategies are adaptable and evidence-based. Additionally, its dementia screening tool combines cognitive assessments with evaluations of biomedical and psychosocial factors to provide individualized risk insights.
Kairoi specializes in AI-powered Decision Support System (DSS)
Nigerian startup Kairoi builds AI-driven platforms that streamline public health insurance management and improve community health worker effectiveness. The platforms automate processes like claims adjudication, benefits enrollment, and fraud detection. They also provide real-time data analytics to support effective and informed decision-making.
Moreover, the platform reduces administrative burdens and improves patient care delivery by integrating digital workflows and decision-support tools.
10. Administrative Automation
Administrative tasks consume a major portion of healthcare budgets. According to the 2024 CAQH Index, automating administrative workflows could save the US healthcare industry up to USD 20 billion annually by reducing manual processes and errors.
Administrative burdens contribute to healthcare professional burnout. Athenahealth’s 2025 survey finds a 22% decrease in the number of doctors considering leaving the profession each week. This links the drop to the use of AI tools that reduce administrative tasks.
Automated systems reduce errors in medical records and billing. The European Journal of Computer Science and Information Technology finds that automation reduces administrative errors by 30% and increases clinical procedure efficiency by 25%.
Further, generative AI automates the creation of clinical notes and reduces the time clinicians spend on documentation. AI-driven scheduling tools optimize appointment bookings, reduce no-shows, and improve resource utilization. For example, European hospitals adopt Inquira Health‘s AI scheduling system to decrease missed appointments and streamline patient intake.
AI also improves EHR systems by automating data entry and analysis, leading to better patient care. Microsoft‘s Dragon Copilot leverages voice dictation and ambient listening to automate note-taking and clinical documentation. Ambient AI scribe tools also transcribe and summarize patient-clinician interactions in real time to reduce documentation time.
Further, AI streamlines the insurance claims process, reduces errors, and improves reimbursements. For instance, Charta Health automates patient chart reviews and identifies missed codes to prevent claim denials. This leads to an increase in revenue and efficiency. AI also analyzes patient data to offer diagnostic and treatment suggestions, improving clinical outcomes.
Cavell AI creates a Co-Pilot for Medical Administration
Belgian startup Cavell provides an AI-powered co-pilot that automates medical documentation for healthcare professionals. The co-pilot captures and transcribes consultations in real-time. It also generates structured notes, referral letters, and patient summaries while adhering to the clinician’s preferred style and format.
Moreover, the AI-enabled co-pilot extracts standardized medical codes and translates clinical language into patient-friendly terms. Further, Cavell prioritizes data security and privacy by storing data locally and maintaining strict GDPR compliance.
Wellora develops an AI Assistant for Clinicians
Australian startup Wellora offers an AI-powered clinical assistant that automates administrative workflows across the full patient care cycle. The startup’s tools include PatientAssist, ConsultAssist, TriageAssist, ScribeAssist, DecisionAssist, TreatmentAssist, and CareAssist.
These modular tools integrate with existing systems to streamline symptom intake, triage, documentation, decision support, and post-visit communication. Moreover, each module addresses a specific phase of care. For example, PatientAssist compiles pre-consult data and ScribeAssist transcribes in-consult conversations into structured notes.
Discover all AI Trends, Technologies & Startups in Healthcare
AI is reshaping healthcare through decentralized computing, digital twins for real-time modeling, and federated learning that protects patient data. Edge AI is also enabling on-device diagnostics, while multimodal systems combine genomic, environmental, and lifestyle data for deeper insights. These advances accelerate clinical trials, drug discovery, and decision-making in critical care. Together, they redefine the speed, precision, and fairness of healthcare delivery.
The Healthcare AI Trends & Startups outlined in this report only scratch the surface of trends that we identified during our data-driven innovation & startup scouting process. Identifying new opportunities & emerging technologies to implement into your business goes a long way in gaining a competitive advantage.